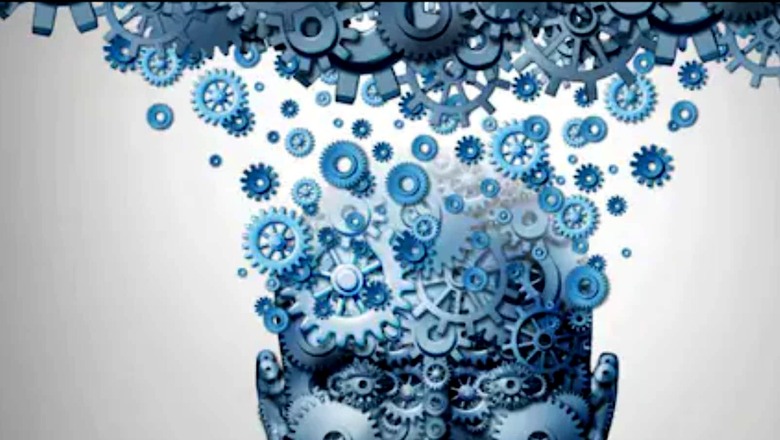
views
Without sacrificing precision, physicists have used artificial intelligence to condense a difficult quantum problem, that formerly required 100,000 equations, down to just four equations. The findings, which were published in the September 23 edition of Physical Review Letters, have the potential to change how scientists analyse systems with multiple interacting electrons. The methodology may also help in the development of materials with desired qualities like superconductivity or use in the production of renewable energy if it is adaptable to other challenges, reported Phys.org.
The intimidating quantum physics problem relates to the movement of electrons on a lattice that resembles a grid. There is interaction when two electrons are present at the same lattice location. With the use of this setup, also known as the Hubbard model, scientists can discover how electron behaviour gives rise to desirable phases of matter, such as superconductivity, wherein electrons move through a material without resistance. The model is also used to test novel methods before they are applied to more complex quantum systems.
The study’s lead author, Domenico Di Sante, an assistant professor at the University of Bologna in Italy and visiting research fellow at the Flatiron Institute's Center for Computational Quantum Physics (CCQ) in New York City, said, “We start with this massive object of all these coupled-together differential equations; then we're using machine learning to transform it into something so small you can count it on your fingers.”
Using a concept known as a renormalization group is one method of investigating a quantum system. This mathematical tool is used by physicists to examine how the behaviour of a system, such as the Hubbard model, varies when researchers alter properties like heat or consider the characteristics on various scales.
It took several weeks for the machine learning algorithm to train, which necessitated a significant amount of processing capacity. The good news, according to Di Sante, is that they can modify their programme to address various issues without having to start from scratch now that it has been coached. To gain new insights that could otherwise be challenging for physicists to understand, he and his colleagues are also examining exactly what machine learning is “learning” about the system.
The key question to be answered is whether the new method can be applied to more complicated quantum systems, like materials in which electrons interact across great distances. Di Sante adds that the technique has intriguing applications in cosmology and neuroscience, as well as other disciplines that deal with renormalization groups.
Read all the Latest Buzz News and Breaking News here
Comments
0 comment