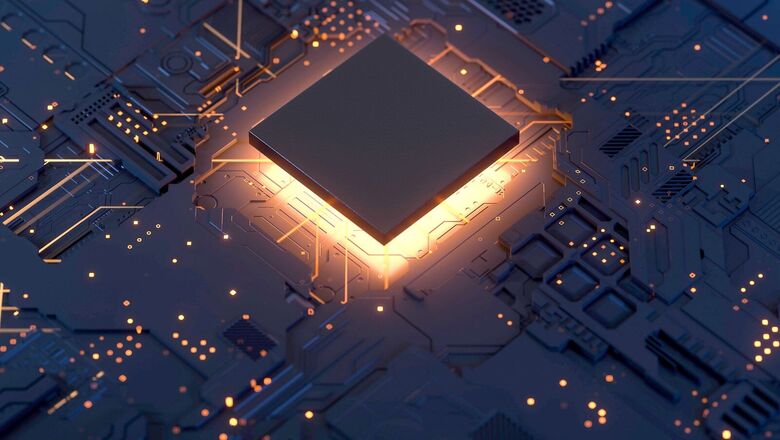
views
The rise of AI applications (from virtual assistants in our homes to facial recognition programs tracking criminals) relies on hardware as a core enabler of innovation. Two main activities enable AI applications: training and inference. The training phase would involve exposure to large data sets to get the algorithm right. At the inference level, however, algorithms would need to respond faster (no time to contact the cloud) to the situation than to store more data. This explains the reliance of the training layer on the cloud and the inference layer on edge/ in-device computing — both of which have varied demands on AI hardware.
When developers are trying to improve training and inference, they often encounter roadblocks related to the hardware, which include storage, memory, logic, and networking. By providing next-generation accelerator architectures, semiconductor companies could increase computational efficiency or facilitate the transfer of large data sets through memory and storage. Specialised AI hardware can make it much better suited to handling the vast stores of big data that AI applications require. With hardware serving as a differentiator in AI, semiconductor companies will find greater demand for their existing chips, but they could also profit by developing novel technologies, such as workload-specific AI accelerators.
As per estimates by McKinsey, AI could allow semiconductor companies to capture 40-50 percent of the total value from the technology stack (the best opportunity to come by in decades). AI-related semiconductors will showcase a growth of about 18 percent (compared to 2 percent of non-AI) annually over the next few years — five times greater than the rate for semiconductors used in non-AI applications. By 2025, AI-related semiconductors could account for almost 20 percent of all demand, translating into about $67 billion in revenue.
A Case for a National AI Hardware Policy
There has been a gradual shift in the semiconductor market, moving from general-purpose to application-specific chipsets. The idea behind an application-driven chip is mainly the ability to perform the same function repeatedly and effectively. AI-enabled hardware fits into the space and is specific to the training algorithm.
The Ministry of Electronics and Information Technology (MeitY) invited applications under the Chips to Startup (C2S) programme for academia, R&D institutions, startups and MSMEs to develop prototypes of application-specific semiconductor chips. It also seeks to train VLSI and embedded systems engineers to design ASICs and FPGAs.
As per a market research report by Markets and Markets, the demand for AI-related and application-specific semiconductors is valued at $7.6 billion in 2020 and is likely to reach $57.8 billion by 2026, at a CAGR of 40.1 percent during the forecast period. The rise in the share of ASICs, GPUs and other applications in the world semiconductor market means that AI hardware is gaining momentum in terms of revenue and importance in the global space. Critical sectors such as space, defence and telecommunications are now on the path to custom hardware with AI capabilities.
AI-enabled hardware can be categorised into two major product categories based on the AI algorithm components: training and inference chipsets. Regarding cost-benefit ratios, the Indian semiconductor manufacturing dream will benefit from investing in AI inference chips fabs rather than display fabs and other chips. There is a cost and requirement gap between the fabrication of AI-enabled chipsets, such as training or inference accelerators and typical semiconductor ICs (both leading and trailing edge). The power, data crunching and memory requirements for manufacturing AI training hardware are more than that of AI inference chips. Specific licensed software to design training hardware also increases the costs of production.
Hence, AI inference chips are the best bet for India when building its ecosystem. Unlike trailing and leading-edge nodes, which need significant capital investments in advanced computing and AI training chips, India can focus only on large-scale inference chip development and manufacturing to make a mark in the AI hardware domain. While AI training hardware has been concentrated with a few firms such as NVIDIA, inference chipsets are low-cost and readily available products designed and manufactured domestically due to their software.
A crucial aspect of the need to focus on AI hardware is the decline in traditional Arm architecture designs to handle high AI workloads. Though ARM holdings released their v9 microarchitecture focusing on AI (Cortex series) in 2020, it remains a costly, licensed and proprietary-based architecture.
Since RISC chips deal with a smaller, less complex set of instructions (relegating most work to software instead), more space is left for adding AI capabilities. Amid existing cost pressures on miniaturisation and packing more capability on a single chip, alternative architectures (like RISC-V) are preferred for integrating high-level AI algorithms with semiconductor chips.
RISC-V is growing in terms of acceptance (due to it being open source with zero licence or royalty fees) as well as the maturity of its ecosystem (the rapid development of compilers and verification tools). The Indian government also launched the ‘digital RISC-V’ initiative, which showcases the tilt towards reducing dependencies on licensed architecture.
With Arm processor cores less feasible to handle AI algorithm training and inference at an adequate level and India pushing towards alternatives such as RISC-V that can benefit AI integration, the focus on AI hardware is imperative currently.
How should India start?
One, build a dedicated trailing edge gab for the large-scale manufacture of inference chips. The government’s ‘Scheme for setting up Semiconductor Fabs in India’ as part of the 2021 semiconductor package provides financial incentives to build fabs from 28 to 65 nm. Trailing edge fabs require less investment and can be set up faster to start production. With trailing edge nodes sufficient for AI hardware production, a 45+ nm fab in the country can be used for the large-scale production of AI inference chips. A priority can be a public-private partnership for a trailing edge fab dedicated to handling AI hardware production.
Two, funding and supporting open-source projects related to AI hardware design is critical. With parallel computing design languages dominated by few firms and their proprietary codes, the government can support (along the same lines as RISC-V) open-source projects to design AI training hardware. OpenAI’s Triton language has been deemed a credible alternative to NVIDIA’s CUDA. US’s DARPA has also developed Real-Time Machine Learning (RTML) to develop ASICs tailored for running ML operations. One such project for the government to focus on could be IISc’s ARYABHAT-1 (Analog Reconfigurable Technology And Bias-scalable Hardware for AI Tasks) — a type of chipset especially helpful for AI-based applications — or those that require massive parallel computing operations at high speeds.
Three, expanding the existing policy schemes to include AI hardware would be a start. The government has also initiated schemes such as the ‘Scheme for setting up Compound Semiconductors Facilities’ and ‘Design Linked Incentive (DLI) Scheme’ to build a domestic semiconductor ecosystem. Research on compound semiconductors like Gallium Nitride (GaN) and Silicon Carbide (SiC) to integrate AI can be kick-started as part of the existing scheme. The scope of the DLI scheme (especially the deployment-linked incentive aspect) can also be broadened to include parallel computing languages and other design aspects related to AI hardware.
With the rise of Artificial Intelligence (AI) and its applications across multiple sectors, there is also the need for better and more efficient computational hardware to handle AI algorithm workload. India must take advantage of the opportunity to make a mark in the low-hanging sector of AI hardware when it has the necessary strength.
Arjun Gargeyas is an IIC-UChicago Fellow and a Consultant at the Ministry of Electronics and Information Technology (MeitY), Government of India. The views expressed in this article are those of the author and do not represent the stand of this publication.
Read all the Latest Opinions here
Comments
0 comment